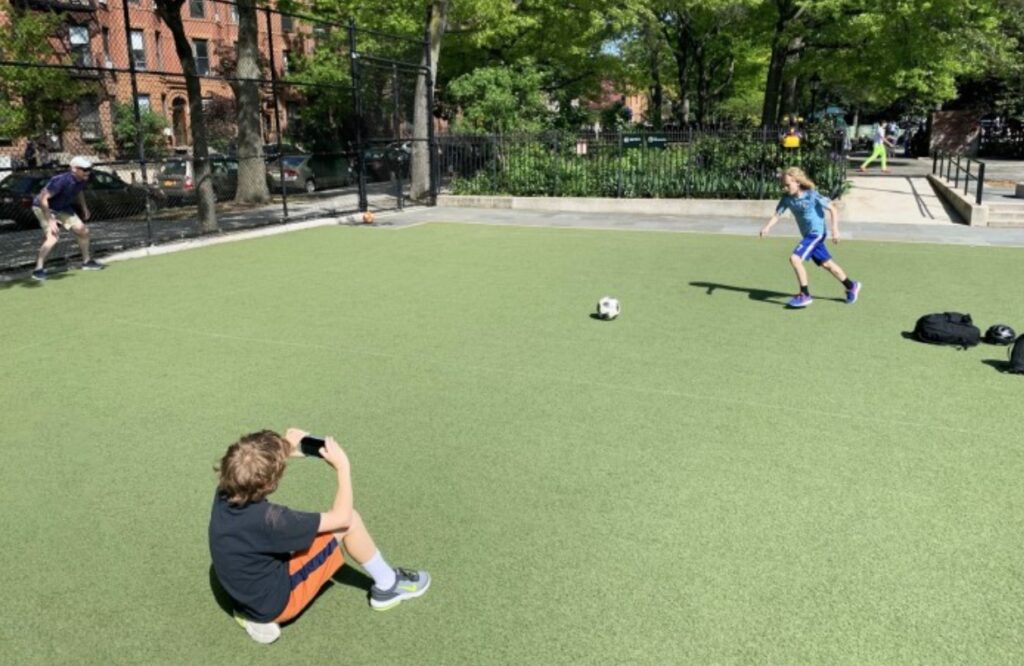
photo image: Eric LoPresti
The other day I user-tested an impressive piece of technology on a field in Brooklyn and got a surprising result. The technology, an artificial intelligence (AI) -assisted prototype, uses computer vision and machine learning (CV+ML) to provide automated skill recommendations for youth team sports. I had engaged a rag-tag team of ten- and eleven-year-olds to install the app on their mobile devices and make videos of each other performing various moves and techniques.
The app represents an impressive, even futuristic stack of technology: a high-speed camera shooting at 240fps combined with a suite of AI algorithms which, together, compared each player’s silhouette against millions of pre-recorded moves, a process called ‘pose estimation’. Even though I grew up programming and have twenty years of experience designing digital technology as a UX architect, watching this combination of real-world interactivity and sophisticated biometrics still feels like something close to magic.
This test had me a bit concerned though. We had spotty connectivity at the park, the lighting kept changing, potentially confusing the CV, and on that day everyone’s phone seemed to be half-broken. The best/worst part: testing with kids. Children tend to be very unforgiving and will quickly discard as “boring” any new tech not perfectly aligned to their immediate purpose. After months of working on this app, we were about to find out: Would they like it?
Turns out I needn’t have worried. The tech performed as designed: each time a junior player performed a technique, the app analyzed the video, automatically gave it a score and displayed a helpful tip on how to improve. Soon the kids were trading their phones back and forth, asking each other to “video me!” in an escalating attempt to beat each other’s scores.
They did more than get it. After an initial gee-whiz moment, the young players folded this magical futuristic technology into their playing like it had been there all along. No one asked, “How does it work?” or “How’d you do that?”. They did like it, but more important: they’d use it.
Sports Data: Quintessential Emotional Tech
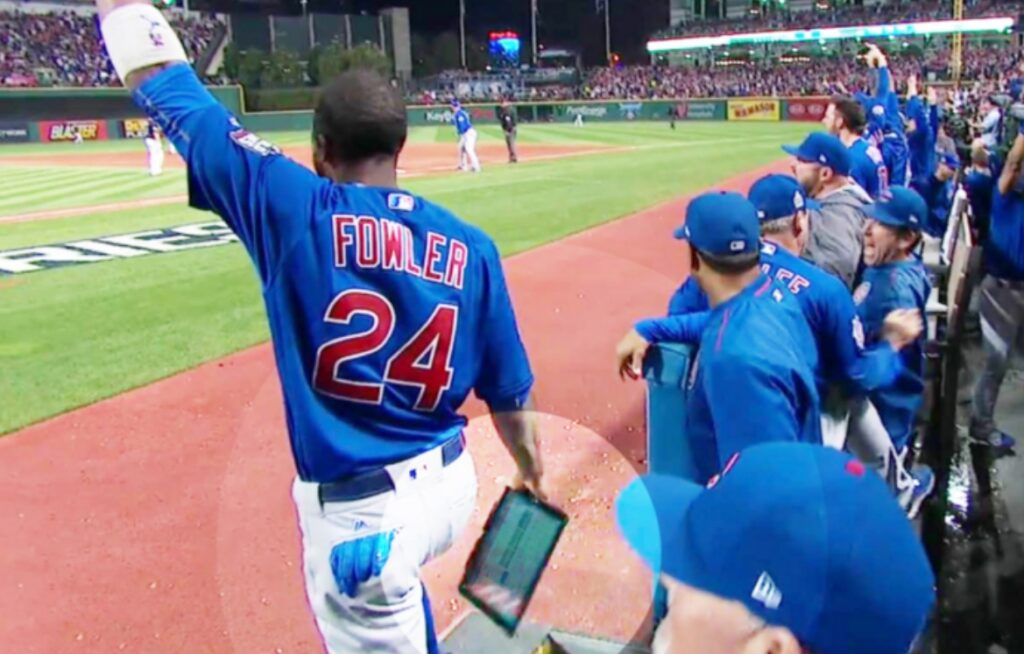
As a user experience (UX) architect I’ve been conceptualizing, designing, and building innovative software for some of the most tech-savvy organizations in the world: Bloomberg, Apple/Major League Baseball, Under Armour, IBM, and the Department of Labor, amongst others. I’ve seen some spectacular technology, including sophisticated AIs that can cull profound insights from the seemingly irrational tangle of human activity. These systems are part of the wave of ‘big data’ which is transforming entire industries, from banking to medicine to engineering, potentially impacting every aspect of our lives.
Recently, while working on a project for Apple/Major League Baseball, I learned something interesting about this wave: Sports data — the application of statistical techniques to professional sports — is way out front. For example, the data collected in a single game of pro baseball is several terabytes, and much of it is available through public APIs, dwarfing comparable sources of consistently-collected biometric data. Plus, sports are emotional. It combines the availability of a wealth of data gathered from a tightly-constrained environment under agreed-upon rules, with the loving, some might say near-irrational devotion of millions of fans. More than any other industry, sports is at the confluence of numbers and feelings.
Baseball in particular is on the leading edge of this wave because of its deep history of counting stats and its geeky superfans tracking athletes’ every move. Two decades ago, a statistical revolution known as “Moneyball”, named after the book by the best-selling author Michael Lewis and further popularized by the eponymous movie starring Brad Pit and Jonah Hill, transformed the game.
Now a new wave of technology powered by the falling price of sensors and sophisticated AI algorithms is transforming the field again, but this time, it will impact a far larger audience. Rather than being constrained to professional sports, this revolution will include amateur sports, personalized physical practices like yoga and working out at the gym, and eventually health and medicine. It represents an inflection point where individuals like you and me will have access to detailed real-time data on our bodies. Call it “Moneyball 2.0.”
Moneyball 1.0: Quants Meet Jocks
In Moneyball: The Art of Winning an Unfair Game (2003), Lewis chronicles how a rigorous approach to statistics changed the game of baseball, ushering in the contemporary era of data-driven sports. The trend had started in the 1970s when obsessive writer-fans like Bill James applied rigorous statistical techniques to baseball, proposing that complex, integrative metrics like a player’s “on-base plus slugging” were better predictors of success than traditional stats like “batting average.” This “sabermetrics” approach was rooted in ready access to stats meticulously hand-recorded from thousands of professional baseball games.
The approach found the apex of its application in 2002, with the struggling performance of the Oakland As. Unable to match the budgets of larger, more powerful competitors, the A’s General Manager Billy Bean employed quantitative statisticians (“quants”) to compose a team of overlooked, lower-priced players based on complex stats. Bean’s innovative and highly-controversial strategy lead to a surprise turnaround, where the A’s won an unprecedented and now-legendary twenty straight games in a row.
That success replicated quickly. Now, most team sports, especially pro soccer, hockey, and football, use some form of Moneyball-style techniques as standard practice, and “Moneyball” is industry slang for “data disruption.” Until recently, though, the technology available to produce these insights was highly specialized and expensive, relegating it to the realm of big-budget professional sports. That’s about to change.
Moneyball 2.0: Personalized Data, Bodies and Feelings
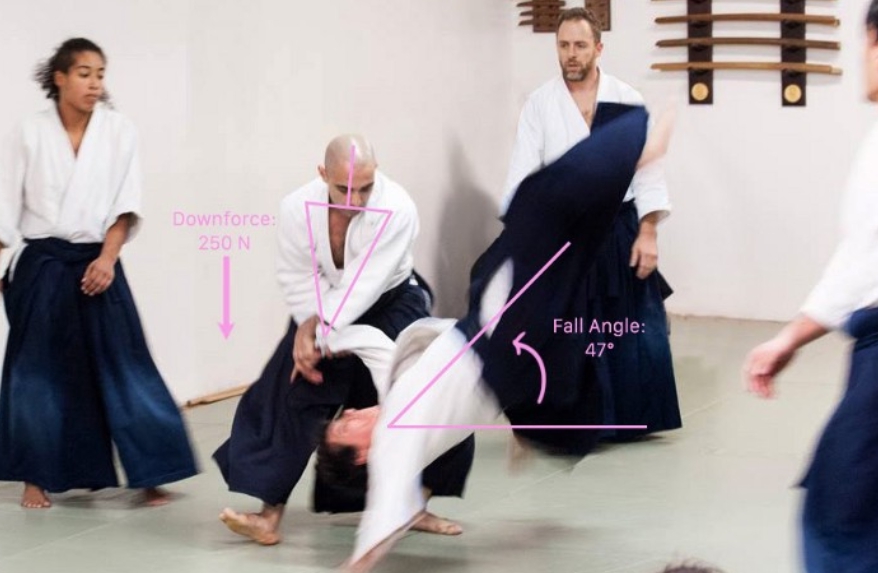
As much as I love baseball, I’m actually more of an individual sports sort of guy. I like biking, swimming, and especially a specific kind of martial arts called aikido. Even before working for MLB, I began to dream about advanced statistics for these kinds of activities: Could I use Moneyball-style data analysis to improve my aikido breakfalls? When would Moneyball be available to me?
That answer will come very soon. In 2019 we are at the intersection of two game-changing curves: the steeply falling price of sensor technology in the form of smartphones and powerful new AI-driven techniques designed to leverage vast troves of data.
MLB required expensive camera installations and military-grade radar to generate advanced stats, but recent generations of mobile phones contain astounding arrays of sensors capable of generating similar information. An iPhone10x, for example, is capable of shooting high-resolution video at 240fps — more than enough to track the position and movement of multiple fast-moving bodies in space. Researchers have even recently used off-the-shelf cameras to enable “contactless” measurement of heartbeat and breathing rate.
Just as impressive is a drop in the price. A decade ago, a motion capture kit (an RF technology with comparable utility as AI-assisted CV) was nearly $20,000. In 2016, improved technology was available for $3000, and today consumers can purchase even better kits for under $500. It’s hard to cite historical pricing data for AI-CV because three years ago that technology was only available in experimental labs.
To leverage this abundance of inexpensive sensor data, newly developed AIs pair CV with ML to crunch data for hidden insights. An example is pose estimation, now open source via codebases like OpenPose and DensePose. Pose estimation enables computers to extract from video data a wireframe model of a human body, including precise measurements of limb positions and joint angles. This unlocks a new universe of biometric stats. For a golf app, for example, one can use pose estimation to determine a player’s form: is their knee bent sufficiently before the swing? Are they following through enough?
Pose estimate isn’t new, but the ability to apply it in real-time to live video data required orders-of-magnitude improvements in algorithms and processing power. Those improvements are now a matter of historical record: new smartphones commonly come equipped with ultra-fast AI-specific processors. What previously belonged to the realm of pro sports is now available to ordinary people like you and me.
Into this nexus comes the prototype I was testing in Brooklyn: an inexpensive app that can provide meaningful insights into complex physical activities in real-time. Thousands, perhaps millions of kids are going to use this in their practice, accumulating a mountain of physical data. AI thrives on large data sets. By segmenting this data by physical demographics — age, height, weight, gender, body type, physical challenges — the AI/ML algorithms can personalize the analytics. An 11-year old girl with a hurt knee is going to be able to learn how to improve her kicking skills by comparing herself to hundreds or thousands of similar players in that category. The insights she gains will be more valuable because the technology bases its analysis, not on a stereotyped silhouette of a generalized player, but her exact body and her precise goals. Welcome to personalized big data.
Sports data will continue to lead this revolution, in part because players are competitive, and Moneyball 1.0 proved that math could be a critical advantage in winning games. On a deeper level, sports, including amateur sports and individual practices, have a special place in human experience. More than just winning and losing, sports are about our bodies, our identities, and our emotions.
These insights will be invaluable to our sense of self. We will be drawn to the benefits of individualized sports data because, ultimately, it’s all about us — we’ll feel it.
The Promise of Personalized Sports Data
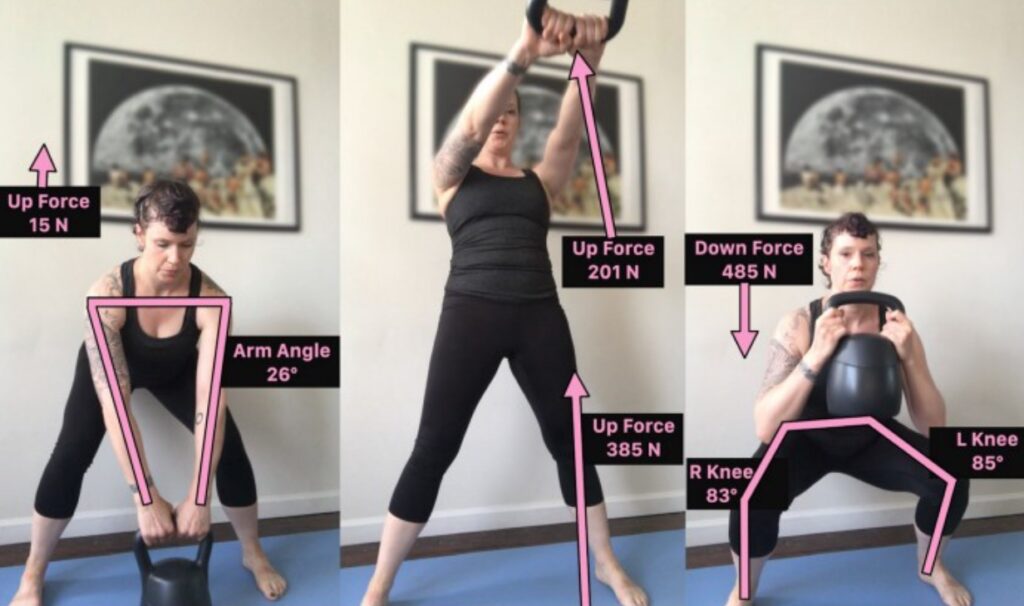
As Moneyball 1.0 transformed professional sports, Moneyball 2.0 will do the same for amateur and non-sport physical activities. Within a decade this technology will extend to health and medicine in ways we can only imagine.
Before this happens, the companies behind these technologies will need to address the critical challenge of data privacy. The same stats which might improve my aikido practice could be used to inform the health insurance industry — for better or for worse. This issue didn’t matter to Billy Bean’s team of professional players, and it’s hardly unique to sports data, but for individuals peering skeptically into the AI-manipulated future, it’s a fundamental issue of trust.
Promisingly, the skyrocketing performance of personal devices may help protect users by giving them physical control over their data. Better cameras and faster processors mean that insight analytics can be done entirely on-device, obviating the need to send individual health stats to a company-controlled server. This physical control, when combined with anonymization and stronger laws around digital privacy, should enable individual users to benefit from the astounding insights of big data without ceding personal control of their intimate data.
Another auspicious trend is that big data can help democratize technology. Moneyball 1.0 was targeted at improving the performance of young, highly athletic, mainly male pro sports players, but Moneyball 2.0 is for everyone else: women, kids, non-athletes, people with disabilities, and perhaps most critically, the large-and-growing aging population intent on extending the quality of their lives. As devices report and aggregate data from tens of millions of bodies in motion, AIs will build ever-more-detailed models for human physiology and diversity, leading to an ever-more-sophisticated and personalized approach to fitness and health.
Within a decade, I expect to realize my dream of applying MLB-style data analytics to aikido. My goal is to use technology-driven insights to adjust and continue my practice as I age, so I can do what I love as long and as well as possible. This dream may sound far-fetched because my chosen practice is so specific, but the core technology already exists. Given my recent experience, a young generation of digital natives already expects this level of smart, data-driven technology to enhance their lives.
The only surprise may be how soon this happens.
Authored By: